As artificial intelligence advances, chatbots have become larger, more sophisticated, and capable of handling complex conversations. With the introduction of bigger chatbots, such as advanced AI models like OpenAI’s GPT series and Google’s Bard, these systems can generate vast amounts of data, providing highly detailed and human-like responses. However, as these chatbots grow in size and complexity, they also tend to produce more inaccuracies, misleading information, or “lies.”
In this article, we will explore why bigger chatbots often tell more lies, examining the root causes behind these inaccuracies and how developers can work to improve the reliability of these systems.
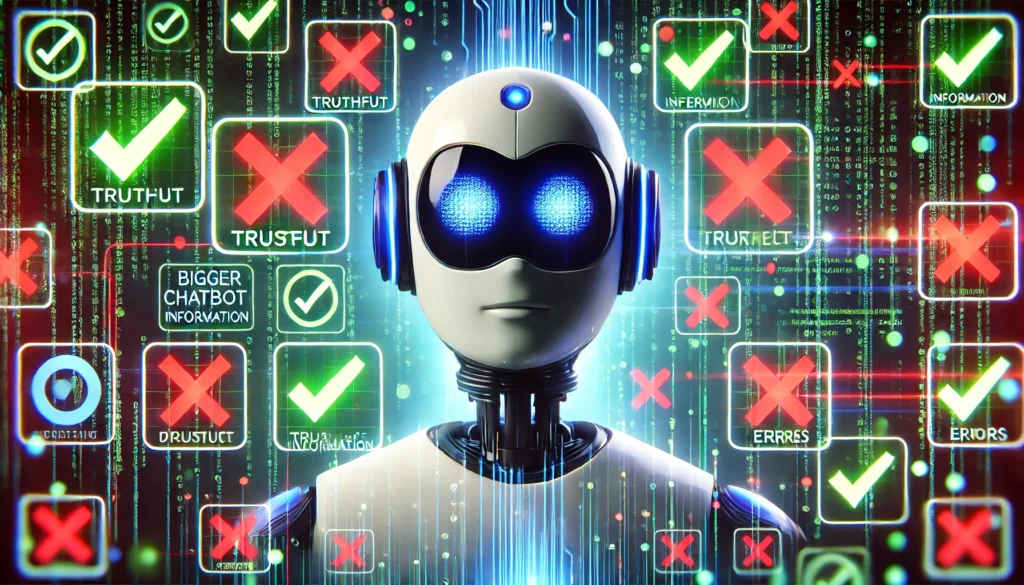
The Complexity of Bigger Chatbots: A Double-Edged Sword
One of the primary reasons why bigger chat-bots tell more lies is the sheer complexity of their architecture. These models, often built using billions of parameters, are designed to mimic human conversation by generating text based on a vast amount of data.
Larger Training Data Means More Possibility for Errors
Bigger chatbots are trained on enormous datasets containing diverse information sources, from scientific papers to social media posts. While this allows them to provide a wide range of responses, it also increases the likelihood of errors. Since the data used for training may include inaccurate or conflicting information, bigger chat-bots can inadvertently generate false or misleading statements.
For instance, if a chatbot is trained on unreliable sources or outdated information, it might present that data as factual. The larger the dataset, the more difficult it becomes to filter out misinformation, increasing the risk of spreading incorrect content.
Overfitting and Hallucination in AI Models
Another challenge with bigger chatbots is their tendency to overfit on the training data. Overfitting occurs when the model becomes too specialized in its training set, leading to biased or overly confident answers. In some cases, chatbots “hallucinate” responses, which means they generate information that appears plausible but is entirely fabricated.
This phenomenon is especially common in larger models, where the complexity of the system can lead to an over-reliance on probabilistic text generation rather than actual knowledge. As a result, bigger chatbots may confidently provide answers that are entirely false, giving users the impression of accuracy when, in fact, the information is incorrect.
Chatbots’ Struggle with Ambiguity and Context
One of the most significant limitations of bigger chat-bots is their inability to fully grasp ambiguity and context in conversation. While these models can process massive amounts of text, they still struggle with understanding nuanced language, intent, and the complexity of human communication.
Lack of True Understanding
Though chatbots are excellent at mimicking human conversation, they lack true understanding of the content they generate. Chatbots operate based on patterns in the data rather than a genuine comprehension of the meaning behind the words. This lack of deeper understanding makes it difficult for them to distinguish between accurate and inaccurate information, leading to the spread of falsehoods.
For example, if a user asks a chatbot a question about a topic with multiple interpretations or unclear details, the chatbot might generate a response that seems confident but is ultimately wrong due to a misunderstanding of the question’s context.
Inability to Handle Uncommon or Niche Queries
The larger a chatbot becomes, the broader its knowledge base, but that doesn’t necessarily translate to accuracy in niche or uncommon areas. When asked a question that falls outside of the chat-bot’s well-trodden training data, it may generate a misleading response simply because it lacks sufficient knowledge in that area. In cases where ambiguity is present, bigger chat-bots often guess or produce incorrect statements rather than admit they don’t have the information.
The Role of User Prompts in Chatbot Lies
Another factor that contributes to why bigger chatbots tell more lies is the nature of the user prompts. Chatbots rely heavily on the questions or instructions given to them, and vague or misleading prompts can lead to incorrect or fabricated responses.
Chatbots Generating Confident but Inaccurate Responses
A common issue with chat-bots is that they are designed to respond with a sense of confidence, even when the response is inaccurate. If a user asks a poorly phrased or ambiguous question, the chatbot may attempt to generate a coherent answer based on its understanding of the prompt, regardless of whether the answer is accurate.
For instance, a user might ask a chatbot a complicated question with multiple layers, and the chatbot might produce an oversimplified answer that does not fully address the nuances. The chatbot’s confidence in delivering these responses can be misleading, causing users to believe the information is correct.
The Challenge of Open-Ended Questions
Open-ended questions are another area where bigger chat-bots can struggle. When given a prompt with no clear right or wrong answer, chatbots often generate responses that align with the most likely or common interpretation of the question. However, this can lead to inaccuracies when the chatbot doesn’t have enough context to provide an informed answer.
This tendency to generate plausible but incorrect responses is a direct result of the chatbot’s reliance on probabilistic text generation, where it tries to predict the next word or phrase based on statistical patterns in the data.
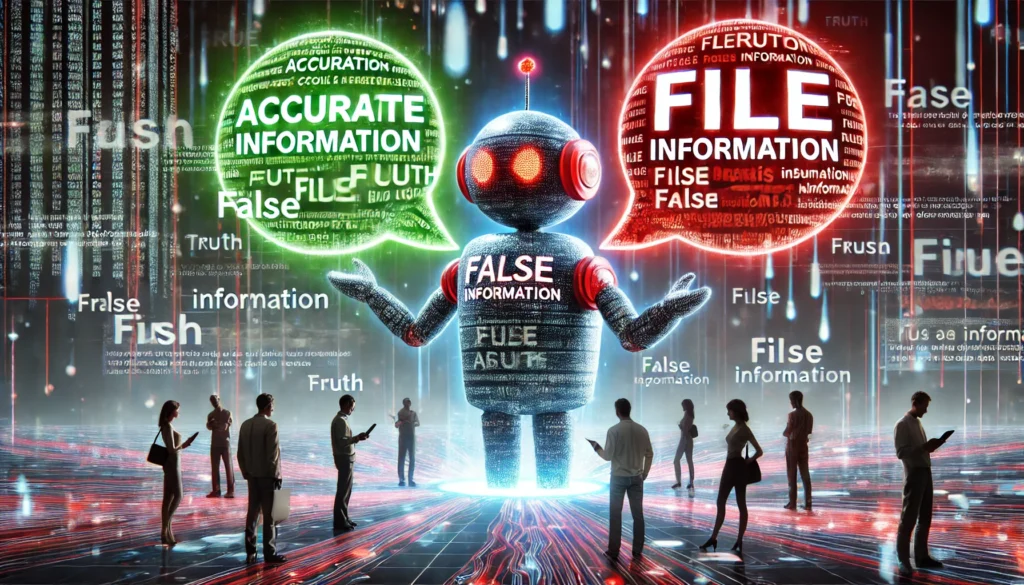
Mitigating Chatbot Misinformation: How to Make Chatbots More Reliable
Despite the challenges of bigger chat-bots telling more lies, there are strategies that can help reduce the likelihood of misinformation and improve the reliability of chatbot responses.
Improved Training Data and Filtering
One solution is to ensure that bigger chat-bots are trained on high-quality, verified data sources. By filtering out unreliable or outdated information during the training process, developers can minimize the risk of chatbots generating false content. Implementing stricter quality control measures during the data collection phase can significantly enhance the accuracy of chatbot outputs.
Incorporating Fact-Checking Mechanisms
Another effective approach is integrating fact-checking algorithms into the chatbot’s architecture. By cross-referencing responses with trusted databases or authoritative sources, chatbots can verify the accuracy of their statements before delivering them to users. This step could prevent bigger chatbots from confidently presenting misinformation.
Transparent Disclaimers
Finally, ensuring that users are aware of the limitations of bigger chat-bots is crucial. Clear disclaimers about the possibility of inaccuracies in responses can help manage user expectations. Encouraging users to verify information from trusted sources, especially when dealing with critical or factual queries, can reduce the impact of potential falsehoods.
Conclusion: Balancing the Benefits and Risks of Bigger Chatbots
While bigger chatbots offer enhanced capabilities, they also come with the risk of generating more misinformation due to their complexity, reliance on probabilistic data, and struggle with ambiguity. As these systems continue to evolve, it’s important to recognize their limitations and take steps to mitigate inaccuracies. By improving training data, incorporating fact-checking tools, and encouraging responsible use, developers and users alike can better navigate the challenges posed by bigger chatbots. As AI continues to advance, finding the balance between chatbot intelligence and reliability will be key to ensuring these tools remain useful and trustworthy in the digital landscape.
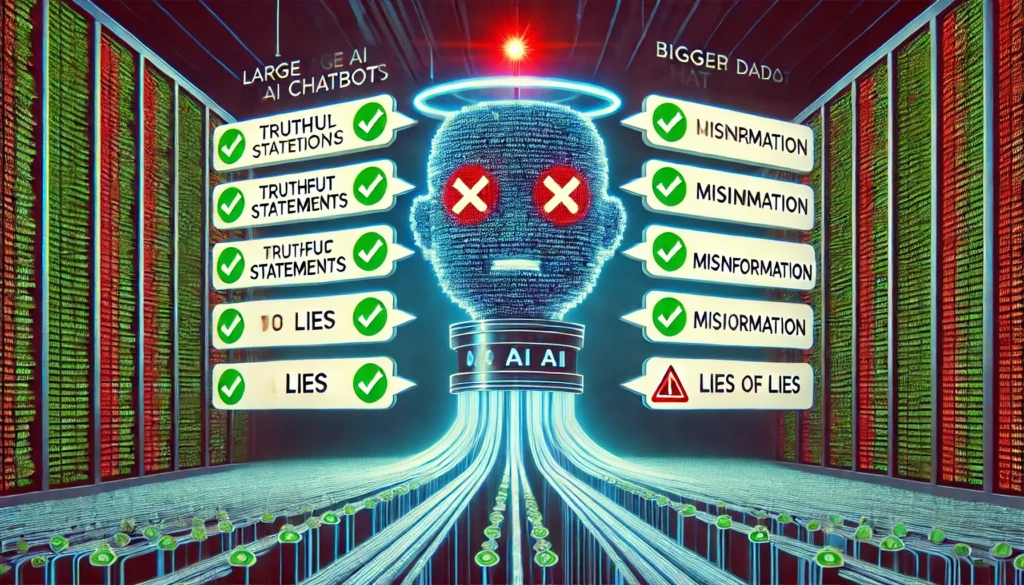
FAQs: Why Bigger Chatbots Tell More Lies
Why do bigger chatbots tend to tell more lies?
Bigger chatbots are trained on vast datasets, which can include inaccurate or outdated information. As these models grow in complexity, the likelihood of generating misleading or false responses increases due to the challenges of filtering large amounts of data and understanding context.
How does overfitting affect chatbot accuracy?
Overfitting occurs when a chatbot becomes too specialized in the training data, leading to biased or overly confident answers. This is common in larger models, where the chatbot might generate incorrect or misleading information while appearing highly confident in its response.
Why do chatbots struggle with ambiguous or nuanced questions?
Chatbots often rely on patterns in data rather than true understanding, so they struggle to grasp ambiguous or nuanced language. As a result, they may generate incorrect answers or oversimplified responses to complex questions because they cannot fully interpret the context.
Can bigger chatbots understand the context of a conversation?
Although bigger chatbots are designed to handle complex conversations, they still lack deep understanding of context. They generate responses based on patterns, which can lead to incorrect or misleading answers, especially in situations where the context is unclear or multifaceted.
How do user prompts contribute to chatbot inaccuracies?
The quality of the user prompt greatly influences the chatbot’s response. Vague or poorly worded prompts can lead chatbots to generate misleading or inaccurate information because they base their answers on incomplete or ambiguous input.
Why do chatbots sometimes “hallucinate” information?
Larger chatbots can sometimes “hallucinate” by generating information that appears plausible but is entirely fabricated. This happens because chatbots rely on probability-driven text generation rather than factual knowledge, making it difficult to discern between true and false information.
How can chatbot lies be minimized?
Chatbot inaccuracies can be minimized by improving the quality of the training data, filtering out unreliable sources, and incorporating fact-checking algorithms. These steps help reduce the likelihood of the chatbot generating false or misleading content.
Are bigger chatbots less reliable than smaller ones?
Not necessarily, but bigger chatbots are more prone to misinformation due to their complexity and the vast amount of data they process. Smaller models may have a narrower scope but can be more focused and potentially less likely to spread false information.
Can chatbots admit when they don’t know the answer?
Most chatbots are designed to provide answers confidently, even if the information is incorrect. However, developers are working on mechanisms that allow chatbots to acknowledge gaps in their knowledge and prompt users to seek additional information from reliable sources.
What steps are developers taking to improve chatbot reliability?
To make chatbots more reliable, developers are enhancing training data quality, integrating real-time fact-checking mechanisms, and promoting transparency through disclaimers. These steps aim to reduce the spread of misinformation and improve the overall accuracy of chatbot responses.